TECHNOLOGY FOCUS
Everything from running shoes and lawnmowers, to windmills, robots and cities are being connected under IoT, Internet of Things. IoT holds great promises of things that alert on emerging issues, that are self-healing, become intuitive, and that avoid unscheduled stand stills. But this will require the machines to become smart, or we will be overwhelmed with a data tsunami that we manually have to monitor and supervise.
That is why Machine Learning goes hand in hand with IoT. Machine Learning helps connected things to automatically learn its normal state and behavior, which enables detection of anomalies, attribution of what has the largest impact on performance, simulation of scenarios, and even prediction of future events.
The intelligence must be personalized for each connected device, which is best done by processing all real-time data instead of old blunt averages. This is why Edge Computing is a prerequisite for IoT, where the processing capacity of the connected device is utilized for Machine Learning.
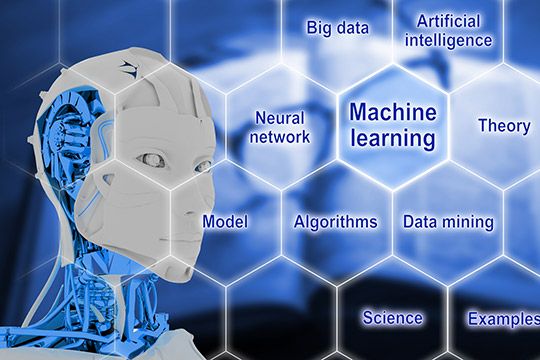
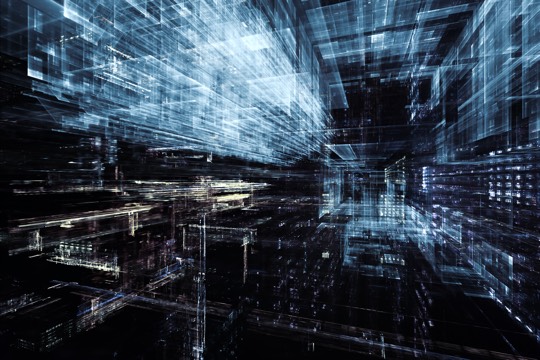
COURSE CONTENT
This course moves quickly from the theoretical basics of Machine Learning via different techniques, IoT requirements and terminology, to hands-on experimentation.
To benefit most you should bring a laptop computer with WiFi capability and your browser of choice. Everything else is provided on site.
WHO SHOULD ATTEND
The course is intended for engineers. Some experience in programming is a benefit, Python especially but not mandatory.
No previous Machine Learning or Data Science experience is required.
The course is favorably combined with course no 102 IoT, Internet of Things - The Next Big Disruption, where we over two days explore use cases and business benefits of utilizing the skills acquired in this course.
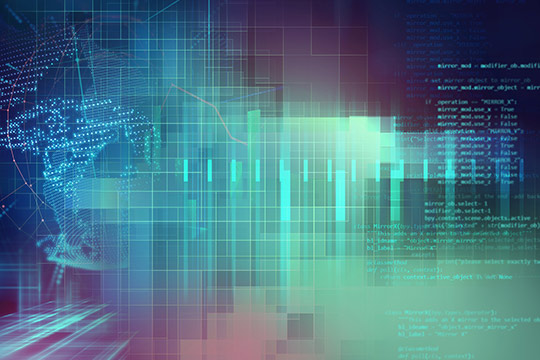
Day 1
- Introduction
- Edge Computing
- Machine Learning
- Classification and Regression
- Data, Training, Evaluation
- Techniques: Decision Trees
Day 2
- Recap from day 1
- Hands-on Machine Learning utilizing Ekkono and Python
- Model Trees
- Random Forest
Day 3
- Machine Learning Functionality for IoT
- Prediction and Forecasting
- Simulation and Optimization
- Attribution/Variable Importance
- Anomaly Detection
- Machine Learning Techniques for IoT
- Accuracy vs CPU vs Memory
- Online vs. Batch Training
- Time Series Prediction for IoT
- Data Modelling
- Evaluation
- Hands-on Exercises on Machine Learning