TECHNOLOGY FOCUS
When moving from 5G to 6G/7G communication networks there will be need for more sophisticated network optimization tools with respect to both computational speed and efficiency of optimization algorithms.
It looks like that Quantum Computing (QC) technology offers significant improvements in both of these aspects. Due to inherent parallelism in the processing of quantum information, unpresented increases in computation speed are anticipated. Similarly a number of algorithms developed within the umbrella of quantum technology, like quantum search algorithms (QSA) developed for big data analysis, could be used to help us with more efficient optimization of communication networks.
In the past, most of the time we would end up with a formulation of the optimization problem that would need exhaustive search through all combination of the system parameters. This would be unacceptable solution when it comes to complexity and as a consequence we would be looking for simplifications that would on the other hand reduce the performance. By combining the advantageousness of both, unprecedented increase of computational speed and efficiency of QSA we will be able to significantly improve the optimization algorithms for network, deployment and optimization.
For these reasons, the focus of this course will be on discussing the principles of QC and detailed presentations of possibilities of using QSA in communication networks.
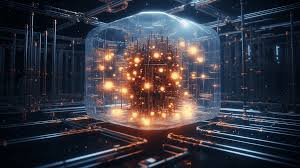
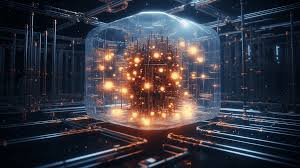
COURSE CONTENT
In order to cover the focus of the course described above, the content will include Fundamentals of Quantum Communications like Qubit system, Geometrical (2-D, 3D) representation of the Quantum States Quantum Gates, Tensor Computing.
Potential Enablers for Quantum Communications like Quantum Entanglement, Hardware Capacity, Key Distribution, Quantum Decision and Game Theory. QC-Assisted Communications like Multi-User Detection/Transmission, Indoor Localization for mmWave and VLC, Joint Routing and Load Balancing and Channel Estimation and Detection.
In addition the course will cover Overview of Quantum Learning Methods like Supervised And Unsupervised QML, Quantum Reinforcement Learning and a number of related topics. Finally, the course will cover a number of practical examples of applications of QML in anticipated 6G/7G networks like: Multi-Objective Optimization and Routing Optimization , Massive-IoT and Big Data Analytics, Security and Privacy, Harmonization and Interoperability of Networks, Configurable Multi-Antenna Systems, Optical, mmWave, and TeraHz Communications, Tiny-Cells and Cell-Free Communications etc.
For more information see the detailed content below.
WHO SHOULD ATTEND
Participants with background in either networks planning, design, deployment and control or networks/internet economics should benefit from participation.
All application examples are focused on 5G and beyond networks and participants with general background in networks will be able to follow the course. This includes researchers, students and professors in academia as well as industry, networks operators, regulators and managers in this field.
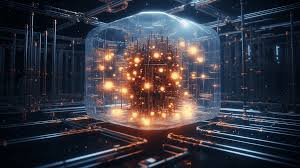
1. Fundamentals of Quantum Communications
Why Quantum Computing? The ever-reducing transistor size following Moore’s law is approaching the point, where the so-called quantum effects become prevalent in the transistors’ operation. This specific trend implies that quantum effects become unavoidable, hence rendering the research of quantum computation (QC) systems an urgent necessity. In fact, a quantum annealing chipset is already commercially available from D-Wave1.
Apart from the quantum annealing architecture, the so-called gate-based architecture, which relies on building computational blocks using quantum gates in a similar fashion to classical logic gates, is attracting increasing attention due to the recent advances in quantum stabilizer codes, which are capable of mitigating the de-coherence effects encountered by quantum circuits. In terms of implementation, D-Wave’s most recent model, namely D-Wave 2000Q, has a total of 2000 qubits, while IBM Q Experience, which relies on the gated-based architecture, has currently only 20 qubits in total. However, IBM has recently announced their plans for delivering a 50 qubit gate-based quantum computer by 2020. Once quantum computing becomes a commercial reality, it may be used in wireless communications systems in order to speed up specific processes due to its inherent parallelization capabilities.
While a classical bit may adopt either the values 0 or 1, a quantum bit, or qubit, may have the values |0>, |1>, or any superposition of the two, where the notation |·> is the column vector of a quantum state. If two qubits are used, then the composite quantum state may have the values |00>, |01>, |10> and |11> simultaneously. In general, by employing b bits in a classical register, one out of 2b combinations is represented at any time. By contrast, in a quantum register associated with b qubits, the composite quantum state may be found in a superposition of all 2b values simultaneously. Therefore, by applying a quantum operation to the quantum register would result in altering all 2b values at the same time. This represents the parallel processing capability of quantum computing.
In addition to superior computing capabilities, multiple quantum algorithms have been proposed, which are capable of outperforming their classical counterparts in the same categories of problems, by either requiring fewer computational steps, or by finding a better solution to the specific problem. In this course, we will focus our attention on the employment of quantum algorithms in classical communication systems, which is nowadays referred to as quantum-assisted communications.
Qubit system
Geometrical (2-D, 3D) representation of the Quantum States
Discrete Variable (DV)/Continuous Variable (CV) Systems
Quantum Gates, Tensor Computing
Quantum Algorithms
Grover's Quantum Search Algorithm (QSA),
Dürr-Høyer QSA
Boyer-Brassard-Hoyer-Tapp QSA
Shor's Algorithm.
Applications of Quantum Communications
Quantum Channel Capacity.
Potential Enablers For Quantum Communications
Quantum Entanglement
Quantum-Dot Cellular Automata
Quantum Hardware Capacity
Quantum Key Distribution
Quantum Decision Theory
Quantum Game Theory
Quantum-Proof Randomness Extractors.
QC-Assisted Communications
Quantum-Assisted Multi-User Detection
Quantum-Aided Multi-User Transmission
Quantum-Assisted Indoor Localization for mmWave and VLC
Quantum-Assisted Joint Routing and Load Balancing
Quantum-Assisted Channel Estimation and Detection.
2. Fundamentals of Quantum Machine Learning
This section revisits the ML methods in the context of quantum-assisted algorithms for ML and the QML framework. Quantum principles based on emerging computing technologies will bring entirely new modes of information processing. An overview of supervised, unsupervised and reinforcement learning methods for QML is discussed in this segment of the course. Special attention will be paid to Spiking Neural Networks motivated by the research results in neuroscience, promising several orders of magnitude energy savings and their Q-equivalent. Life Long Learning concept will be also covered, solving the problem opf algorithm reinitialization for every new learning task.
1) Overview of Quantum Learning Methods
a: Supervised And Unsupervised QML
b: Quantum Reinforcement Learning
2) Generative and Discriminative QML Models
3) Quantum SVMs and ANNs
(support vector machine and artificial neural networks)
4) Perceptron
5) Spiking Neural Networks
6) Life Long Learning
3. Quantum Networks (and 6G/7G)
Quantum Network Protocols
Summary of the analytical tools
Quantum Link Layer Protocol
Reinforcement Learning Based QN Protocols
QN STABILITY
Dynamic QN PROTOCOLS
Q-Internet
Q-Satellite networks
Elementary Link Generation with Satellites
Implementation Aspects of cv Satellite QN
CV QUANTUM KEY DISTRIBUTION
Fundamentals of CVQKD
Security of CVQKD protocols
Composable security proof for cv QKD
QKD OVER SUBOPTICAL BANDS:
QUANTUM vs POST QUANTUM SECURITY
POST‐QUANTUM CRYPTOGRAPHY
Overview of Post-Quantum Cryptosystems
QUANTUM CRYPTOGRAPHY
Discrete Variable Protocols
Continuous‐Variable QKD
One‐way CV‐QKD protocols
Two‐way CV‐QKD protocols
Theoretical Models of Security
IMPLEMENTATION EXAMPLES OF cv QKD
qubit PHYSICS
Superconducting Qubits
Qubit gates using the spin states of coupled single-electron quantum dots
Quantum Logic by Polarizing Beam Splitters
Quantum Gates Implemented by Trapped Ions
https://www.wiley.com/en-us/Artificial+Intelligence+and+Quantum+Computing+for+Advanced+Wireless+Networks-p-9781119790310
https://www.ins-netgroup.com/wp-content/uploads/2022/08/cover-2-.pdf
https://www.ins-netgroup.com/wp-content/uploads/2022/08/cover.pdf
https://www.ins-netgroup.com/uncategorized/series-7g-wireless-networks-report-310719493-quantum-coding-and-criptography/
https://www.ins-netgroup.com/uncategorized/series-7g-wireless-networks-report-310719492-implementation-aspects-of-quantum-computing/
https://www.ins-netgroup.com/uncategorized/series-7g-wireless-networks-report-310719491-continuous-variable-quantum-key-distribution-over-wireless-networks/
https://www.ins-netgroup.com/uncategorized/series-7g-wireless-networks-report-090819823-quantum-networks/
https://www.ins-netgroup.com/uncategorized/series-7g-wireless-networks-report-090819822-quantum-computing-with-continuous-variable/
https://www.ins-netgroup.com/uncategorized/series-7g-wireless-networks-report-090819821-quantum-machine-learning/
including lecture notes for applications of Quantum Computing in Complex Networks, covering large scale networks, information,
technological, biological, quantum computational chemistry, neuroscience, and brain study …
https://www.ins-netgroup.com/uncategorized/quantum-computing-in-complex-networks-volume-1/
https://www.ins-netgroup.com/uncategorized/quantum-computing-in-complex-networks-volume-2-2/